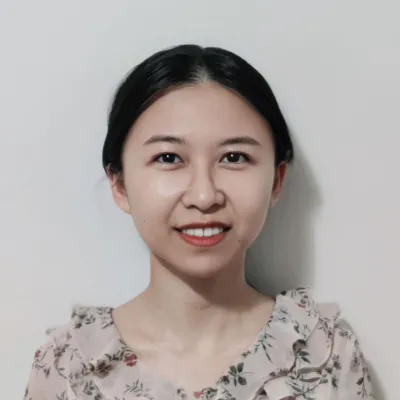
Qiuxiao Chen
Contact
Bio
Dr. Qiuxiao Chen holds a Ph.D. in CS from the Utah State University. Her Ph.D. research focused on computer vision of autonomous driving, 3D Object Detection and Self-attention Mechanism. Her research interests encompass (1) autonomous driving systems utilizing deep learning and (2) image processing for intelligent parking systems. Dr. Chen is actively involved in the development of autonomous driving systems that utilize multiple cameras, lidar sensors and employ deep learning algorithms.
Education
Ph.D. Computer Science, Utah State University, US, 2023.
B.S. Electrical Engineering, Zhejiang University, China, 2018.
Courses/Teaching
CSE 1300- Introduction to Data Science
CSE 5700- Compilers
Research and Teaching Interests
Dr. Chen's interests are in the areas of deep learning, autonomous driving 3D object detection, and Bird’s-Eye-View (BEV) segmentation. Nowadays, the capability to generate a real-time perception and have awareness of the surroundings is crucial for autonomous vehicle navigation. In order to drive safely, autonomous systems must be able to extract spatial object information and understand the semantic meaning of the environment in real-time. Only with such comprehension, can a self-driving vehicle maneuver safely while obeying traffic rules. Motivated by this, Dr. Chen's research aims to improve autonomous driving by increasing the network’s ability to extract spatial object and environment semantic information.
3D object detection: 3D object detection in autonomous driving is a critical technology that enables self-driving vehicles to perceive their surroundings in three dimensions, using sensors like LiDAR, radar, and cameras. This process identifies and locates objects around the vehicle, facilitating navigation, path planning, and collision avoidance by providing a detailed understanding of the environment. The technology faces challenges such as sensor fusion and environmental conditions, yet it remains essential for the safety and efficiency of autonomous vehicles.
BEV segmentation: BEV (Bird's Eye View) segmentation in autonomous driving is a technique used to generate a top-down, 360-degree view of the vehicle's surroundings by segmenting various elements of the environment from sensor data. This process involves classifying areas around the vehicle into different categories, such as roads, pedestrians, vehicles, and obstacles, based on the data collected from onboard sensors like cameras and LiDAR. BEV segmentation helps in improving the understanding of spatial relationships and the layout of the environment, crucial for navigation, planning safe paths, and executing complex driving maneuvers with precision.